COMMENT: AI, whether we realise it or not, is affecting all our lives in significant ways today, writes PropAI founder and tech commentator Antony Slumbers.
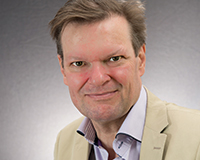
From monitoring our credit card usage for fraud, to filtering our email for spam, to recommending what to watch on Netflix, to recognising our friends in photographs on Facebook, to flying our planes for 95% of the time, to enabling self-driving cars. For many day-to-day processes it is becoming pervasive behind the scenes.
AI will become pervasive within real estate as well. The extraordinary new capabilities of computers to understand the world around them, to see, hear and read as well as humans, are sure to provide the foundational tools that allow us to build a better-built environment.
But how do you need to think about AI in real estate?
First you need to understand what AI is good for, and just as importantly what it is not. You need to analyse your workflows and business processes to see where you can leverage what AI is good at. You also need to consider which of these use cases is commonplace and repeatable within your own business and/or applicable to many of your customers.
Look for use cases
You need to look for use cases where you have or can obtain large quantities of data, and where there are clear metrics by which you could judge success.
And finally, if you have all of the above, is AI something that will create significant value?
Over the past five years AI has developed rapidly in three key areas: computer vision, voice recognition and natural language processing. Taking computer vision as an example, in 2013 the ImageNet competition winner (ImageNet runs annually and quantifies the capabilities of different systems) had an error rate of 11.3%. In 2017 that had come down to 2.2% (For reference, humans rate at 5%).
Similar improvements apply to neuro-linguistic programming (NLP) and voice recognition. In practical terms these three skills have gone from useless to useful and this is why we have seen the rapid rise of driverless cars and the explosive rise of Amazon’s Alexa.
AI’s abilities
Today, AI is very good at understanding what is happening in pictures and videos; understanding people using language; creating content (auto-generated commentary, extracting data from reports, news etc); automating processes; optimising complex systems; and making predictions.
Essentially, anything that is structured, repeatable or predictable can (and most probably will) be automated. Think of all of this as pattern recognition and you’ll get to grips with what is possible.
Once your thinking is clear about what AI is good at, you need to look for use cases. What tasks do you or your company perform that are structured, repeatable or predictable? Last year the RICS reported that 88% of the tasks surveyors perform are vulnerable to being automated within 10 years. In 2016 McKinsey said that 49% of all tasks people are paid to perform globally are capable of being automated by currently demonstrable technology.
List them out. What do you do that follows a pattern in some way, either because they involve linear processes, or because you know that with these inputs you know you can produce those outputs. You’ll find workflows or processes from across the entire lifecycle of real estate, from planning, to design, construction, leasing, occupation and management and on to portfolio analysis and investment sales or acquisition.
Key questions
What imagery or video content do you deal with, or what movements around space would be useful to track? What customers do you interact with and how much do you know about them? What reports do you have to generate and how are they put together; could these be automated? How do you handle questions from customers; do these follow a pattern?
Once prepared, you can move to the third stage. Take your list and give each item two scores of 1 to 10. How common is this task in your business, and how common is it outside your business? Now we need to look at data. How much of it about each task do we have? Here you should think of the four Vs: volume, variety, velocity and veracity. How much data do we have, from how many different sources, how fast is it being generated (as per seconds, hours, days, months, years) and how accurate is it?
You need to think about correlation not being causation and apply that to your data. You need to think in terms of “with these data points I could arrive at this conclusion”. For example, if I knew exactly how occupiers of my building moved around, I could optimise the AC and lighting. Or, if I knew these characteristics of an investment, I could match them against these characteristics of an investor.
Thinking about AI in real estate involves understanding new technologies, looking for use cases, determining what use cases are core and ongoing or transitory, getting to grips with data, determining what you need and whether you have it or can get it, and then homing in on where real value lies. Once you have a structured way to think about this new world, things do tend to fall into place quicker than you imagine.
Utilising AI is as much a mindset as a technology.